The Blueprint for a Data Governance Program
Employee productivity might be summed up by the expression “The last thing I need in my day is_________.”
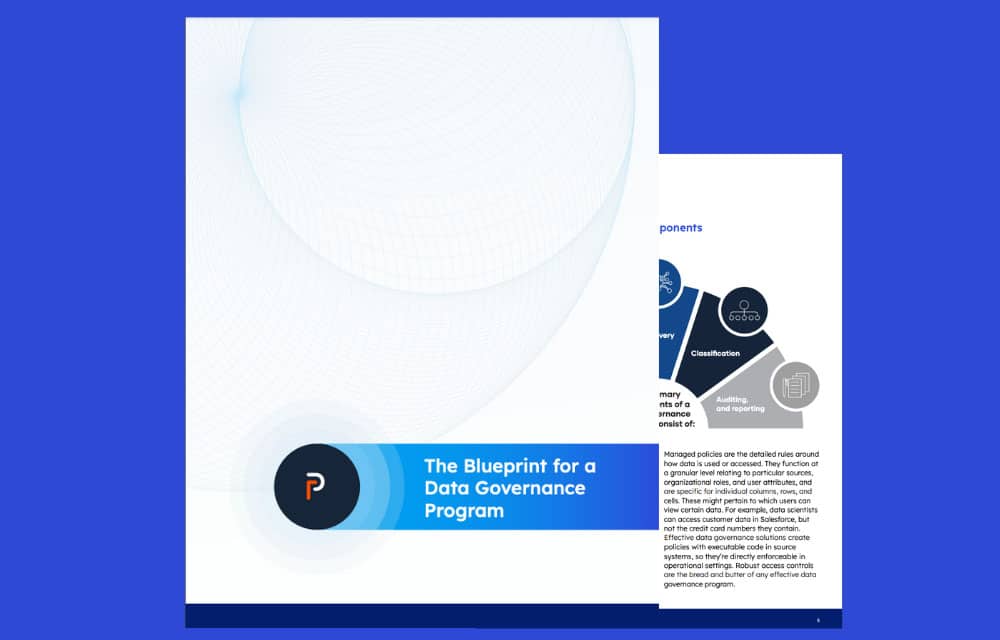
1 The Blueprint for a Data Governance Program
Most organizations realize data governance is necessary - if not indispensable - for mitigating data risk while sustaining its long term value. However, there’s still a fair amount of ambiguity around what exactly data governance is and how to best implement it.
By definition, data governance is an enterprise-wide initiative for formalizing the processes, people, and protocols for using data. It reduces risk while increasing data’s ultimate value: consistent reusability for achieving mission critical objectives. Data governance is a strategic approach for creating unassailable trust in data throughout the enterprise.
However, it’s far more difficult to succeed in implementing a data governance program than it is to define this term. There are many more companies trying to create governance programs than there are those who have done so successfully.
Gartner indicates 80 percent of companies attempting to scale digital business will fail because they don’t have a modern approach to data and analytics governance.
New Vantage Partners revealed that organizations are struggling to become data driven, as only 26.5% have achieved this end, while less than 20 percent have established a data culture. The main reason for these woes is often data governance is an afterthought. A company’s business objectives aren’t based on data governance, but rather on solving specific business problems related to strategic business objectives such as cryptocurrency, electric cars, etc.
Oftentimes, companies don’t prioritize data governance until they reach a sufficient maturity level in terms of revenues, customer base, data quantities, and sources. Then, governance outputs—such as gaining the visibility of where sensitive data resides, ensuring the right people have access to the right data for the right purposes, or reinforcing corporate responsibility by responding to data and privacy regulations—become paramount.
Moreover, many fledgling governance initiatives are hampered by multi-tasking. SMBs usually don’t have dedicated governance personnel. A small credit union, for example, has perhaps 20 employees managing multiple tasks. If someone’s doing any data stewardship or data governance work, they usually have several other responsibilities preventing them from concentrating on it.
2 Business Drivers and Data Governance Justification
It’s critical for businesses to recognize and believe in the potential of their data. A governance program inspires such trust by assuring end users about what possibilities their data supports. Consequently, there are several business drivers that justify the need to implement data governance programs. Most pertain to fostering an organizational culture of trust in data. In this regard, secure data access is a preeminent requisite for users to trust their data. Data security encompasses several dimensions, such as ensuring data’s controls are current, consistent, complete, and reproducible. If marketers are attempting to mine customer data for insights, they need to know it’s trustworthy and customers have given their consent to use their data for promotional purposes.
Risk management is another driver, particularly because of the rapid technological advancements that have accelerated over the past several years. Technological developments are close to outpacing those of humans, which creates trust gaps when the former enables people to do so much that not all of it’s legal. Technology is going so fast that people can do more than ever before. The question is: should they? Popular social media platforms didn’t collect tons of graph data to break laws; no one initially told them they couldn’t and that data contains plenty of customer insights. In these situations, oftentimes
organizations didn’t know what they couldn’t do because of a lack of resources for a creditable governance program.
The continual emergence of data privacy regulations typifies the need to implement governance for regulatory compliance. With GPDR, CCPA, and various state level mandates being enacted, companies need governance just to assess what data relates to which regulation. There are also industry-specific regulations (for finance and healthcare, for example), and data sovereignty issues in the cloud about where data reside.
Additionally, data is the raw material for data science. This discipline requires high quality data without regulatory complications, which is only possible with effective governance. Employing Artificial Intelligence and machine learning to understand customer behavior and improve customer satisfaction is another compelling driver. These analytics capabilities are the new data products enabling organizations to understand, anticipate, and fulfill customer needs in an era in which customer experience is a competitive differentiator. AI and ML predictions can reduce customer friction so companies can go from being
product-oriented to customer-oriented.
Therefore, a good AI strategy needs a good data strategy.
3 Data Governance Components
Guidelines are the specifics about what employees and business partners can and can’t do with a company’s data. Data access is one of the strictest guidelines; it’s enabled for some users on some datasets and restricted for others.Guidelines are frequently based on regulatory requirements, data governance councils’ input, and collaborations with business end users. Data residency and permissible purpose are examples of guidelines that keep users “within the authorized lines” of what they can do with data.
Managed policies are the detailed rules around how data is used or accessed. They function at a granular level relating to particular sources, organizational roles, and user attributes, and are specific for individual columns, rows, and cells. These might pertain to which users can view certain data. For example, data scientists can access customer data in Salesforce, but not the credit card numbers they contain. Effective data governance solutions create policies with executable code in source systems, so they’re directly enforceable in operational settings. Robust access controls are the bread and butter of any effective data governance program.
Governance programs must also have capabilities for evaluating, monitoring, enforcing, and validating users’ access to data. Data discovery is the starting point of these programs and is the capability to identify sensitive elements in data throughout the enterprise. Organizations know they have sensitive data; the problem is finding it. Traditionally, time-consuming manual approaches were used, which were ineffective. Automated data profiling capabilities are much better. Classification is the next step in the process, and is the ability to categorize data according to definitions, regulations, use cases, and more. Classifications are part of data cataloging functionality that provides a central means of denoting where data is, what it’s regarding, who owns it, and other vital information.
The next step in the process is the ability to define and enforce access control policies. These policies can be based on users’ organizational roles, attributes, and other such things. Access control policies also involve auditing chronicles of who accessed data and how, for what reason, what operation was performed on it, where it was sourced, and other particularities. Ideal solutions let administrators easily get answers to these questions, which are invaluable for demonstrating regulatory compliance, assessing risk, and understanding which regulations pertain to data.
4 Stakeholders and Dependencies
Regardless of which data governance approach is employed – top down, bottom up, or a hybrid – the stakeholders for governance programs are usually the same. These include C-level executives, data owners, data stewards, and end users. It’s imperative to get the approval and participation of the C-level for program success. Since these executives fund these programs, continuing them hinges on tangible indicators of success to validate their worth to the C-level. Since these executives are directly responsible for corporate adherence, the primary incentive for these stakeholders is regulatory responsiveness—regardless that they’re the ones funding governance programs—so organizations aren’t jeopardized by new regulations.
Data owners are the top personnel in business units, such as a VP of Finance, for example. Their chief area of interest is increasing their department’s effectiveness and business value by using data. Data stewards are generally assigned to respective business units by data owners. Stewards actively participate in governance programs; their responsibilities include developing an understanding of data and its usage, granting data access, ensuring policies are met, ensuring sufficient guidelines are in place, and monitoring operational activities. This role is oftentimes multitasked.
There are two types of end users: internal and external ones. Internal users are data analysts or data scientists. Data scientists want to create innovative solutions without transgressing policies, regulations, and data governance protocols. External end users are customers or business partners. They’re motivated by the growing scope of data privacy rights for things like subject access requests or honoring data contracts. Subject access requests enable data subjects to ask firms what information they have about them. These requests often spur executives into action for governance programs. The rationale is if firms can’t fulfill this request for their own customers, they’ll have tremendous difficulty doing so for others.
5 Implementation
There’s a two-step process for initiating implementation of a data governance program. The first is identifying exactly where an organization’s data is at the source level. Pinpointing what data are in which sources involves mapping how the organization itself functions. Oftentimes, a company’s business units indicate how it’s mapped. An insurance company, for example, has divisions for products, policies, sales, and field representatives. These units are the major business areas that the leadership of the business naturally thinks of as fundamental. When assessing this information, it’s key to not only consider what information or data is generated by these different departments, but also what data they need to function optimally.
Once organizations determine where exactly their various data is by mapping how their businesses function, the second step is that they must craft a conceptual data model or subject area model to reveal exactly what that data is. Specifically, this step revolves around the business meaning ascribed to various data. Time-honored approaches involving ontologies and taxonomies are invaluable for articulating the world view of a specific business unit as expressed through data. These methods identify the particular terms, semantics, and nomenclature business users understand, which are generally more helpful than conceptualizing data in arcane terms only IT teams comprehend.
6 Data Governance Best Practices
Since the goal of data governance programs is to foster unconditional trust in data, best practices for such programs center around a dedicated data access management strategy. That strategy naturally incorporates aspects of what data is in which sources and what it actually means to the various business units that interact with it—as identified in the initial implementation process above. This information is theefoundation upon which an effective data access management strategy is built. Once that’s been solidified, firms can engage in data discovery mechanisms (which are another hallmark of creditable data governance solutions) to delineate exactly where their data is. Data access management strategy best practices for data governance include:
9
These staples of an access management strategy are analogous to parking in a garage so someone can’t steal the tires on your car. The reality is fences make good neighbors, which is exactly what guidelines are for valuable enterprise data.
Another best practice is to ingrain data stewardship into the process of developing the business, instead of trying to create a separate data governance stack later. By getting started with this aspect of data governance early on, organizations cultivate a culture of—and respect for—data governance that’s essential to sustain these programs for the long term. Aligned with this
concept is the best practice of selecting an adaptive architecture that flexibly expands to include different sources.
Most businesses leverage data sources that are external to the enterprise and frequently found in hybrid cloud, multi-cloud, poly cloud, or edge computing environments. The ability to seamlessly connect to these resources via a distributed architecture that pushes governance and security policies directly into these various cloud services is priceless for successfully governing them. Moreover, data stewards are central to ensuring this architecture supports underlying data governance objectives.
7 Pitfalls To Avoid
The aforementioned Gartner and New Vantage Partner statistics strongly support the notion that most organizations either never complete their governance programs or fail to do so successfully. Consequently, the first pitfall to avoid is over-thinking the various considerations involved in creating a successful data governance program. Organizations should adhere to the best practices in this document for implementing data governance, but they should also prioritize taking action over the time-honored ‘analysis paralysis’ syndrome. Governance should be part of an overall data management program.
Ideally, the various steps, tips, and practices detailed above should be implemented in a non-intrusive, non-invasive manner. It may be helpful to contextualize these different measures by positioning them to relevant stakeholders (whose organizations may already be doing some data governance work) in order to avoid duplication of effort and resources. Following the above advice about establishing a data governance program only formalizes those measures, makes them consistent, and repeatable throughout the enterprise—instead of in different departments or silos.
Multitasking is another pitfall organizations should make a point to avoid. Governance programs work best when employees who have other responsibilities don’t take on additional ones for data governance. As previously indicated, this situation happens far too frequently, making governance programs difficult to scale and ineffective. It’s way more advantageous to have dedicated employees for the various data governance positions.
The final pitfall to avoid is attempting to unify enterprise data and sources for the purpose of improving data governance. Doing so invokes the single source of truth conundrum, in which the holy grail of data-driven practices or analytics is to physically consolidate all data into a single repository to implement data governance mechanisms there. There are numerous approaches predicated on achieving this objective, which involves cloud data warehouses, conventional ETL, data lakes, and data lakehouses.
Nonetheless, this is often an exhaustive task requiring several months or years to finish. During that time business requirements and data sources change, nullifying any value from this endeavor. Also, by trying to drive down time to insight with these holistic platforms, organizations may inadvertently accelerate time to a lawsuit—without the proper governance in place.
8 A Modern Prerequisite
A data governance program is a vital prerequisite for obtaining long term value from any significant investment in data-driven processes. It’s silently transitioned from something that’s only necessary for the most mature organizations to something allorganizations must embrace—or suffer the regulatory, reputational loss, and customer dissatisfaction consequences
This paper outlines the specific business drivers, various stakeholders, components, implementation processes, best practices, and pitfalls for a data governance program. Organizations would be well to internalize these tips and strategies for establishing a longstanding data governance program that augments the recurring business value data itself produces for the enterprise
Learn more at privacera.com
Related Articles
Join The GBI Impact Community
Sign up to make an impact and hear about our upcoming events
By registering anywhere on the site, you agree with our terms and privacy policy