The world’s largest Apache Kafka® and Apache Cassandra® migration?
https://www.instaclustr.com/blog/the-worlds-largest-apache-kafka-and-apache-cassandra-migration/
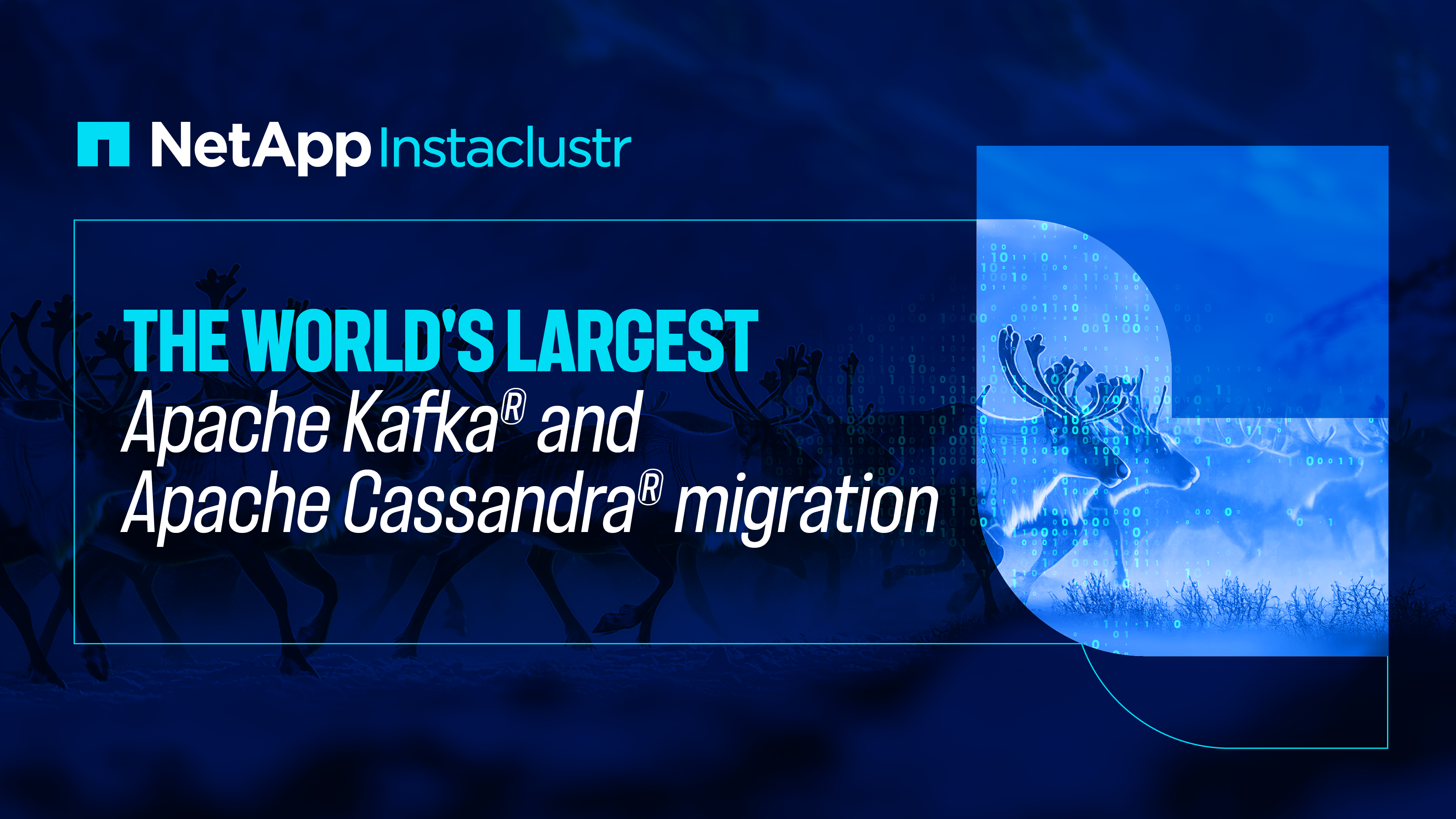
Here at NetApp® Instaclustr, we pride ourselves on our ability to migrate customers from self-managed or other managed providers with minimal risk and zero downtime, no matter how complex the scenario. At any point in time, we typically have 5-10 cluster migrations in progress. Planning and executing these migrations with our customers is a core part of the expertise of our Technical Operations team.
Recently, we completed the largest new customer onboarding migration exercise in our history and it’s quite possibly the largest Apache Cassandra and Apache Kafka migration exercise ever completed by anyone. While we can’t tell you who the customer is, in this blog we will walk through the overall process and provide details of our approach. This will give you an idea of the lengths we go to onboarding customers and perhaps to pick up some hints for your own migration exercises.
Firstly, some stats to give you a sense of the scale of the exercise:
- Apache Cassandra:
- 58 clusters
- 1,079 nodes
- 17 node sizes (ranging from r6g.medium to im4gn.4xlarge)
- 2 cloud providers (AWS and GCP)
- 6 cloud provider regions
- Apache Kafka
- 154 clusters
- 1,050 nodes
- 21 node sizes (ranging from r6g.large to im4gn.4xlarge and r6gd.4xlarge)
- 2 cloud providers (AWS and GCP)
- 6 cloud provider regions
From the size of the environment, you can get a sense that the customer involved is a pretty large and mature organisation. Interestingly, this customer had been an Instaclustr support customer for a number of years. Based on that support experience, they decide to trust us with taking on full management of their clusters to help reduce costs and improve reliability.
Clearly, completing this number of migrations required a big effort both from Instaclustr and our customer. The timeline for the project looked something like:
- July 2022: contract signed and project kicked off
- July 2022 – March 2023: customer compliance review, POCs and feature enhancement development
- February 2023 – October 2023: production migrations
Project Management and Governance
A key to the success of any large project like this is strong project management and governance. Instaclustr has a well-established customer project management methodology that we apply to projects:
In line with this methodology, we staffed several key roles to support this project:
- Overall program manager
- Cassandra migration project manager
- Cassandra technical lead
- Kafka migration project manager
- Kafka technical lead
- Key Customer Product Manager
The team worked directly with our customer counterparts and established several communication mechanisms that were vital to the success of the project.
Architectural and Security Compliance
While high-level compliance with the customer’s security and architecture requirements had been established during the pre-contract phase, the first phase of post-contract work was a more detailed solution review with the customer’s compliance and architectural teams.
To facilitate this requirement, Instaclustr staff met regularly with the customer’s security team to understand their requirements and explain Instaclustr’s existing controls that met these needs.
As expected, Instaclustr’s existing SOC2 and PCI certified controls meant that a very high percentage of the customer’s requirements were met right out of the box. This included controls such as intrusion detection, access logging and operating system hardening.
However, as is common in mature environments with well-established requirements, a few gaps were identified and Instaclustr agreed to take these on as system enhancements. Some examples of the enhancements we delivered prior to commencing production migrations include:
- Extending the existing system to export logs to a customer-owned location to include audit logs
- The ability to opt-in at an account level for all newly created clusters to be automatically configured with log shipping
- Allowing the process that loads custom Kafka Connect connectors to use instance roles rather than access keys for s3 access
- Enhancements to our SCIM API for provisioning SSO access
In addition to establishing security compliance, we used this period to further validate architectural fit and identified some enhancements that would help to ensure an optimal fit for the migrated clusters. Two key enhancements were delivered to meet this goal:
- Support for Kafka clusters running in two Availability Zones with RF2
- This is necessary as the customer has a fairly unique architecture that delivers HA above the Kafka cluster level
- Enabling multiple new AWS and GCP node types to optimize infrastructure spend
Apache Kafka Migration
Often when migrating Apache Kafka, the simplest approach is what we call Drain Out.
In this approach, Kafka consumers are pointed at both the source and destination clusters; the producers are then switched to send messages to just the destination cluster. Once all messages are read from the source cluster, the consumers there can be switched off and the migration is complete.
However, while this is the simplest approach from a Kafka point of view, it does not allow you to preserve message ordering through the cutover. This can be important in many use cases, and was certainly important for this customer.
When the Drain Out approach is not suitable, using MirrorMaker2 can also be an option; we have deployed it on many occasions for other migrations. In this particular case, however, the level of consumer/producer application dependency for this approach ruled out using MirrorMaker2.
This left us with the Shared Cluster approach, where we operate the source and destination clusters as a single cluster for a period before decommissioning the source.
The high-level steps we followed for this shared cluster migration approach are:
- Provision destination Instaclustr managed cluster, shut down and wipe all data
- Update configurations on the destination cluster to match source cluster as required
- Join network environments with the source cluster (VPC peering, etc)
- Start up destination Apache ZooKeeper™ in observer mode, and start up destination Kafka brokers
- Use Kafka partition reassignment to move data:
- Increase replication factor and replicate across destination as well as source brokers
- Swap preferred leaders to destination brokers
- Decrease replication factor to remove replicas from source brokers
- Reconfigure clients to use destination brokers as initial contact points
- Remove old brokers
For each cluster, a detailed change plan was created by Instaclustr to cover all of the high-level steps listed above and rollback if any issues arose.
A couple of other specific requirements from this environment that added extra complexity worth mentioning:
- The source environment shared a single ZooKeeper instance across multiple clusters. This is not a configuration that we support and the customer agreed that it was a legacy configuration that they would rather leave behind. To accommodate the migration from this shared ZooKeeper, we had to develop functionality for custom configuration of ZooKeeper node names in our managed clusters as well as build a tool to “clean” the destination ZooKeeper of data related to other clusters after migration (for security and ongoing supportability).
- The existing clusters had port listener mappings that did not align with the mappings supported by our management system, and reconfiguring these prior to migration would have added extensive work on the customer side. We therefore extended our custom configuration to allow more extensive custom configuration of listeners. Like other custom configuration we support, this is stored in our central configuration database so it survives node replacements and is automatically added to new nodes in a cluster.
Apache Cassandra Migration
We have been doing zero downtime migrations of Apache Cassandra since 2014. All of them basically follow the “add a datacenter to an existing cluster” process that we outlined in a 2016 blog.
One key enhancement that we’ve made since this blog–and even utilized since this most recent migration–is the introduction of the Instaclustr Minotaur consistent rebuild tool (available on GitHub here).
If the source cluster is missing replicas of some data prior to starting the rebuild, the standard Cassandra data center rebuild process can try to copy more than one replica from the same source node. This results in even fewer replicas of data on the destination cluster.
Instaclustr Minotaur addresses these issues.
This can mean that in the standard case of replication factor 3 and consistency level quorum queries, you can go from having 2 replicas and data being consistently returned on the source cluster to only 1 replica (or even 0 replicas) and data being intermittently missed on the destination cluster.
The “textbook” Cassandra approach to address this is to run Cassandra repairs after the rebuild, which will ensure all expected replicas are in sync. However, we are frequently asked to migrate clusters that have not been repaired for a long time and that can make running repairs a very tricky operation.
Using the Minotaur tool, we can guarantee that the destination cluster has at least as many replicas as the source cluster. Running repairs to get the cluster back into a fully healthy state can then be left until the cluster is fully migrated, and our Tech Ops team can hand-hold the process.
This approach was employed across all Cassandra migrations for this customer and proved particularly important for certain clusters with high levels of inconsistency pre-migration; one particularly tricky cluster even took two and half months to fully repair post migration!
Another noteworthy challenge from this migration was a set of clusters where tables were dropped every 2 to 3 hours.
This is a common design pattern for temporary data in Cassandra as it allows the data to be quickly and completely removed when it is no longer required (rather than a standard delete creating “tombstones” or virtual delete records). The downside is that the streaming of data to new nodes fails if a schema change occurs during a streaming operation and can’t be restarted.
Through the migration process, we managed to work around this with manual coordination of pausing the table drop operation on the customer side while each node rebuild was occurring. However, it quickly became apparent that this would be too cumbersome to sustain through ongoing operations.
To remedy this, we held a joint brainstorming meeting with the customer to work through the issue and potential solutions. The end result was a design for the automation on the customer-side to pause the dropping of tables whenever it was detected that a node in the cluster was not fully available. Instaclustr’s provisioning API provided node status information that could be used to facilitate this automation.
Conclusion
This was a mammoth effort that not only relied on Instaclustr’s accumulated expertise from many years of running Cassandra and Kafka, but also our strong focus on working as part of one team with our customers.
The following feedback we received from the customer project manager is exactly the type of reaction we aim for with every customer interaction:
“We’ve hit our goal ahead of schedule and could not have done it without the work from everyone on the Instaclustr side and [customer team]. It was a pleasure working with all parties involved!
“The migration when smoothly with minimal disruption and some lessons learned. I’m looking forward to working with the Instaclustr team as we start to normalize with the new environment and build new processes with your teams to leverage your expertise.
“Considering the size, scope, timeline and amount of data transferred, this was the largest migration I’ve ever worked on and I couldn’t have asked for better partners on both sides.”
Interested in doing a migration yourself? Reach out to our team of engineers and we’ll get started on the best plan of action for your use case!
=====================================================================
Listicle: Harnessing managed open source: The future of data infrastructure
URL: https://www.instaclustr.com/blog/harnessing-managed-open-source-the-future-of-data-infrastructure/
Data isn’t just a byproduct of business—it’s the driving force behind smarter decisions, innovative strategies, and future growth. As businesses put more focus on their data strategies, intelligent data infrastructure is becoming essential. This year, open source technologies are set to transform how companies store, process, and analyze their data.
What does this mean for you? It’s an opportunity to stay ahead by leveraging the latest trends in data infrastructure. Whether you’re exploring managed open source solutions or scaling your existing systems, these advancements can help your organization unlock the full potential of your data.
Let’s dive into the key trends shaping the future and how they can empower your business.
1. The rise of unified data storage
Managing massive amounts of data is one thing—managing it efficiently across various environments is another. In 2025, organizations will pursue unified and more intelligent data storage solutions that integrate seamlessly across on-prem, hybrid, and cloud systems. Open source tools will play a pivotal role in this transformation, offering innovation in flexibility and interoperability.
Impact: Unified storage solutions will increase demand for open source communities that actively innovate and improve capabilities for advanced data management. Open source’s flexibility means businesses can customize solutions to meet their exact needs, but with this comes the responsibility of scaling and managing the complexity of these ecosystems to ensure projects remain efficient and sustainable.
2. The resurgence of real-time data processing
The demand for real-time insights is accelerating. Whether it’s detecting fraud, powering recommendation engines, or managing IoT systems, businesses need data processing infrastructure that operates with minimal latency. Open source technologies like Apache Kafka® are already leading the charge, but in 2025, the focus will shift to expanding use cases, improving accessibility, and integrating more cost-effective solutions like tiered storage.
Tiered storage is proving to be a game-changing component, enabling organizations to optimize costs and scale their real-time systems by intelligently managing data. Frequently accessed, high-priority information is stored on faster, premium tiers for immediate access, while less critical data is shifted to more economical, lower-performance tiers. This ensures that real-time systems remain agile and cost-efficient, even as data volumes grow.
Impact: Enterprises will benefit immensely from leveraging open source technologies like Apache Kafka, supported by tiered storage, to build robust, scalable, and efficient data pipelines that meet the demands of modern workloads. By balancing performance and cost, tiered storage not only simplifies maintaining low-latency systems but also makes real-time actionable intelligence more accessible for businesses of all sizes.
3. Privacy-first and distributed data architectures
With stricter data privacy regulations and rising concerns over centralization, open source projects will increasingly focus on privacy-first and distributed data solutions. Technologies like federated learning and decentralized storage (think IPFS and Pachyderm) will allow organizations to process data without moving or exposing it entirely.
In 2025, we’ll see more open source projects designed to enable secure, collaborative data sharing—allowing businesses to analyze data across borders and internal silos while staying compliant with privacy laws. Expect new frameworks for maintaining data sovereignty and preventing breaches while enabling the analysis of sensitive datasets.
Impact: Healthcare and telecommunications industries will likely lead adoption, balancing stringent regulation with the need for data insights. Implementing distributed architectures, however, may require a significant up-front investment.
4. AI-driven search capabilities
AI-driven search capabilities will redefine how businesses extract value from their data. By incorporating advanced natural language processing (NLP) and machine learning models OpenSearch® is enabling highly intuitive, real-time search experiences that adapt to user behavior and uncover deep insights across vast datasets. This evolution aligns perfectly with the demands of modern workloads such as real-time analytics, customer personalization, and operational intelligence, empowering businesses to optimize data infrastructure for speed and seamless access.
Impact: Enhanced search functionality improves operational efficiency by reducing the time spent navigating and retrieving critical information. Simultaneously, the ability to analyze data in real time enriches customer experiences through faster, more relevant service delivery. For organizations leveraging data-driven decision-making, OpenSearch provides a powerful foundation for surfacing actionable intelligence, driving more informed strategies, and unlocking new opportunities for innovation.
5. The evolution of open source data warehousing
Open source data warehouses like ClickHouse® will continue to evolve, offering lighter, faster, and more scalable solutions. In 2025, they’ll compete head-on with proprietary systems, integrating deeper analytics capabilities and machine learning extensions. The trend will shift from businesses simply adopting open source solutions for cost-saving purposes to leveraging them as central components of modern data strategies. We’ll also see enterprise-focused, managed versions of these technologies gaining traction, allowing even smaller businesses to unlock advanced analytics without high overhead.
Impact: Enterprises, which rely on granular operational data, will leverage open source warehouses to drive efficiency and innovation. The challenge will lie in handling large-scale migrations and workforce training to adopt new tools effectively.
6. The re-prioritization of infrastructure modernization
Open source technologies are transforming how critical workloads like VMware, Oracle, and other enterprise systems operate. A key trend for 2025 is enhanced interoperability, with open source technologies—like Kubernetes and Apache Kafka, enabling seamless integration across hybrid environments. This allows legacy systems to connect easily with modern cloud infrastructures, helping enterprises modernize without replacing existing investments.
Another major development is improved scalability. Open source technologies now support dynamic scaling to handle demanding workloads—from large databases to resource-heavy applications. This flexibility helps businesses manage unpredictable demands while maintaining strong performance and efficiency.
Cost-efficiency is also a key advantage, with open source reducing licensing costs and optimizing resources. Additionally, it offers enhanced customization, allowing businesses to create solutions tailored to their specific needs.
Impact: These advancements allow businesses to unlock greater flexibility and performance while continuing to rely on critical enterprise systems like VMware and Oracle. By modernizing legacy workloads and aligning them with cutting-edge open source solutions, organizations can innovate faster, maintain cost control, and remain adaptable in a rapidly evolving data landscape.
Final thoughts
Open source is transforming the way organizations plan their data strategies, delivering cost efficiency, scalability, and innovation all at once. But as data infrastructure grows in prominence, businesses must be prepared to adopt and adapt. Each of these trends—unified platforms, real-time processing, privacy-first systems, AI-driven search, open warehouses, and infrastructure modernization—represents an opportunity to turn data into a powerful asset.
The question isn’t whether open source will drive enterprise innovation in 2025, but rather how fast your organization can leverage these technologies. Those who scale quickly will find themselves better equipped to innovate, deliver value, and stay ahead of the curve.
What open source data infrastructure trends are you excited to explore? The future of data-driven business is taking shape. Are you ready to seize it with a managed open source solution?
Related Articles
Join The GBI Impact Community
Sign up to make an impact and hear about our upcoming events
By registering anywhere on the site, you agree with our terms and privacy policy